AI image processing transforms how we extract value from visual data. These sophisticated algorithms enhance poor-quality images, detect objects, and reveal insights hidden in pixels. From facial recognition to document preprocessing, AI combines computer vision with machine learning to interpret what cameras capture. The process involves data collection, model training with CNNs, feature extraction, and deployment across industries like healthcare and security. The visual data revolution isn’t coming—it’s already transforming your digital world.
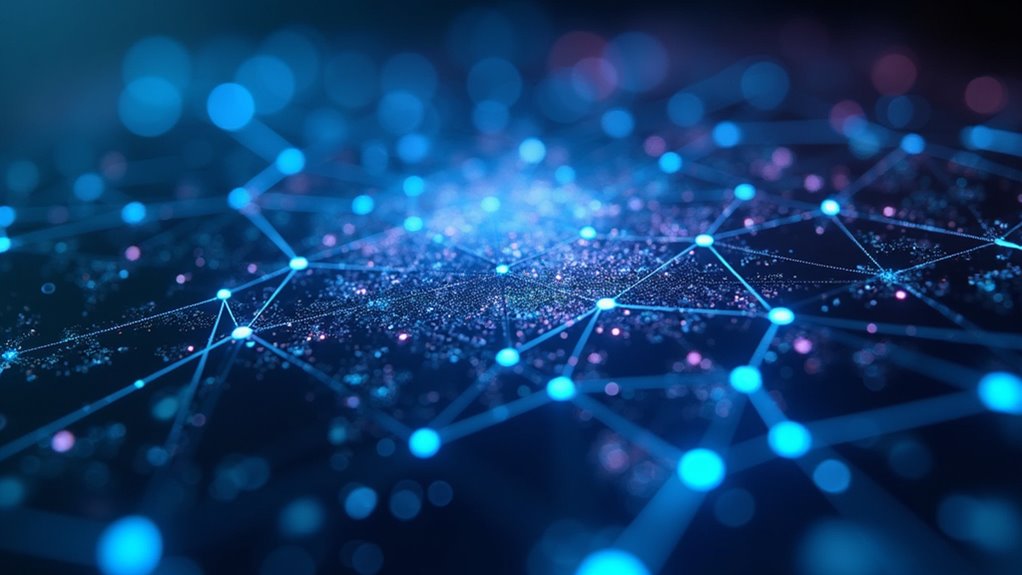
As digital imagery continues to dominate our visual landscape, AI image processing has emerged as a revolutionary force transforming how we interact with visual data. This rapidly evolving technology applies sophisticated algorithms to understand, interpret, and manipulate images in ways previously unimaginable. The combination of artificial intelligence and computer vision has created powerful tools that extract meaningful insights from visual information that would overwhelm human analysts.
Image enhancement techniques represent one of the most accessible applications of this technology. Ever taken a photo in poor lighting? AI can fix that. These algorithms reduce noise, adjust brightness, and improve contrast—transforming mediocre snapshots into crisp, clear images. Document workflows significantly benefit from these enhancements, as preprocessing tasks ensure optimal quality for subsequent analysis.
AI transforms your worst photos into your best, enhancing every pixel with intelligent precision.
Meanwhile, object detection algorithms have become ubiquitous in modern applications. They’re what help your phone recognize faces, allow security systems to identify suspicious activity, and enable autonomous vehicles to distinguish between pedestrians and street signs. Popular platforms like Google Cloud Vision offer comprehensive solutions that can identify objects, detect facial features, and recognize text in images. Don’t underestimate these algorithms; they’re literally changing how machines “see” our world.
The mechanics behind AI image processing follow a predictable pattern: collect relevant data, train models (usually Convolutional Neural Networks), extract features, validate performance, and deploy for inference. This process powers applications across industries—from healthcare professionals using AI to detect diseases in medical scans to security systems identifying potential threats in real-time. Recent research shows that AI-generated imagery can transform personal data into visual representations that enhance meaning-making processes for users seeking to better understand their own information.
The benefits are substantial and growing. Organizations implementing these technologies report increased efficiency, improved accuracy, and significant cost savings. The competitive advantage alone justifies the investment for many businesses. Who wouldn’t want better insights faster?
Looking ahead, AI image processing will continue its rapid expansion. The market is projected to grow exponentially by 2025, with new applications emerging regularly. As algorithms become more sophisticated and computing power more accessible, we’ll see even greater integration of these technologies into everyday life. The visual data revolution isn’t coming—it’s already here, interpreting the world around us one pixel at a time.
Frequently Asked Questions
How Do Privacy Laws Affect AI Image Processing Implementations?
Privacy laws markedly restrict AI image processing implementations through strict data protection requirements.
Organizations must navigate complex legal compliance landscapes that vary by region—GDPR in Europe, CCPA in California, and Australia’s Privacy Act all impose different constraints.
These regulations demand consent, transparency, and data minimization practices when collecting and analyzing visual information.
The privacy implications are substantial, forcing developers to implement privacy-by-design approaches and regular audits to prevent potential breaches or unauthorized surveillance through image analysis technologies.
What Computing Resources Are Needed for Real-Time Image Processing?
Real-time image processing demands robust computing resources.
GPU acceleration provides the parallel processing power critical for handling complex algorithms simultaneously.
Edge computing reduces latency by processing data locally, while cloud computing offers scalable resources for more intensive applications.
Hardware optimization guarantees efficiency through specialized processors like TPUs and FPGAs.
The balance between these resources depends on application requirements—autonomous vehicles need ultra-low latency edge solutions, while less time-sensitive applications might leverage cloud infrastructure’s power.
Can AI Detect Manipulated or AI-Generated Images?
Yes, AI can detect manipulated or AI-generated images through various techniques.
Manipulation detection systems analyze noise patterns, pixel inconsistencies, and compression artifacts that reveal tampering.
Meanwhile, generated image verification tools like BrandWell and Illuminarty use deep learning to spot AI-created content.
Don’t be fooled though – these tools aren’t perfect! As generation technology evolves, detection becomes a constant cat-and-mouse game.
Human oversight remains vital to verify results from these increasingly sophisticated detection systems.
How Are Image Datasets Ethically Sourced for AI Training?
Ethical image dataset sourcing requires obtaining explicit image consent from individuals featured in photos—no shady stuff allowed!
Dataset transparency means clearly documenting where and how images were collected. Companies must also address bias by including diverse populations and scenarios.
Many organizations now employ dedicated ethics teams to review collection practices. The best datasets balance privacy protection with representation, while synthetic data generation offers a promising alternative when real images raise ethical concerns.
What Cybersecurity Risks Exist in AI Image Processing Systems?
AI image processing systems face significant cybersecurity risks, including data breaches that can expose sensitive visual information to unauthorized parties.
These systems often struggle with algorithmic bias, which may perpetuate discriminatory patterns. Attackers can manipulate these systems through adversarial attacks, tricking them into misclassifying images.
Additionally, inadequate access controls create vulnerabilities, while deepfake generation capabilities raise serious authentication concerns.
Organizations must implement robust encryption, regular security audits, and bias testing to protect these increasingly valuable systems.