AI transforms object detection by replacing manual feature extraction with automated deep learning systems. Modern CNNs process thousands of images in seconds, enabling real-time applications in autonomous vehicles, healthcare, and manufacturing. Models like YOLO and SSD balance speed with accuracy, while Feature Pyramid Networks improve detection across various scales. Though environmental factors can challenge these systems, continuous improvements address limitations. The ethical implications of surveillance capabilities deserve serious consideration before implementation begins in earnest.
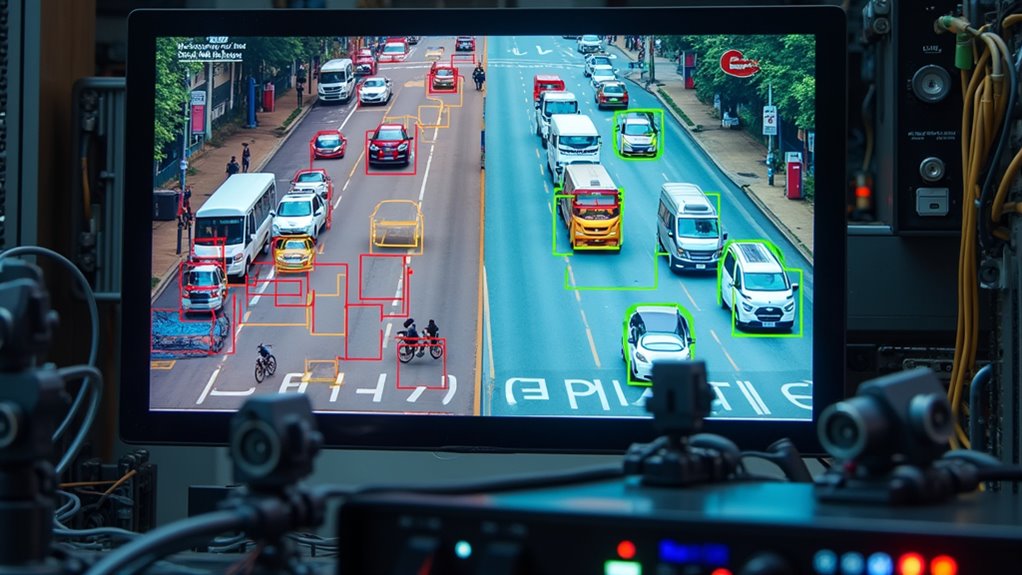
While traditional computer vision relied on manual feature extraction, modern AI-powered object detection has revolutionized how machines interpret visual data. Deep learning models, particularly Convolutional Neural Networks (CNNs), now handle the heavy lifting of feature extraction automatically—no more painstaking algorithm tweaking! The entire pipeline, from data preprocessing to model training, has become more sophisticated yet accessible.
You’d be amazed how these AI algorithms can process thousands of images in seconds, identifying patterns humans might miss entirely. Real-time processing capabilities have transformed industries overnight. YOLO and SSD models don’t just detect objects—they do it blazingly fast while you’re still blinking. This performance evaluation isn’t just academic; it translates to life-saving applications in autonomous vehicles that must detect pedestrians instantly.
Speed isn’t just a luxury in AI object detection—it’s the difference between theory and life-saving reality.
But let’s not kid ourselves about the ethical implications. The same technology that keeps drivers safe can raise serious privacy concerns when deployed in surveillance systems. Popular platforms like Google Cloud Vision offer powerful APIs that developers can integrate into their applications with minimal effort. Application diversity continues to expand across sectors. Healthcare professionals now rely on object detection for analyzing medical images, while manufacturers use it for quality control on production lines. The continuous learning capabilities of these models help them reduce false positives and become more accurate over time.
The technology adapts to varied environments, though environmental factors like poor lighting can still trip up even the most robust systems. Multi-task loss functions help models simultaneously handle the dual priorities of classification and localization for effective object detection. The architecture behind these systems combines convolutional layers with specialized networks like Region Proposal Networks. Feature Pyramid Networks enhance detection across multiple scales—crucial when you’re trying to identify both a building and a bird in the same image.
Newer transformer-based models offer end-to-end processing that previous generations could only dream about. Despite impressive advances, challenges remain. These models demand massive datasets and computational resources that aren’t available to everyone. Finding the sweet spot between speed and accuracy requires careful optimization—you can’t have everything!
As object detection technology continues evolving, balancing its benefits against limitations will remain an ongoing conversation in the AI community.
Frequently Asked Questions
How Much Computational Power Is Required for Real-Time Object Detection?
Real-time object detection demands substantial computational power. High-performance GPUs are typically essential, with models like YOLOv7 requiring significant resources despite their efficiency.
Latency considerations are critical—every millisecond counts! Edge devices need optimized architectures, while server-based systems can leverage more powerful hardware.
The computational requirements vary based on model complexity, desired frame rates, and resolution. For truly responsive systems, dedicated AI accelerators or multi-GPU setups are often necessary.
Can AI Object Detection Work in Low Visibility Conditions?
AI object detection can indeed work in low visibility conditions, but it’s not magic—just smart engineering. Low light performance improves through specialized algorithms that enhance dim images before processing.
Sensor fusion is the real game-changer, combining camera data with radar or LiDAR to “see” through fog, rain, or darkness. Think of it as your eyes and ears working together when you can’t see well.
Modern systems achieve this by cross-referencing multiple data streams simultaneously.
How Are Privacy Concerns Addressed in Surveillance Applications?
Privacy concerns in surveillance applications are addressed through several key approaches.
Data anonymization techniques like blurring faces and license plates help protect individual identities.
Organizations implement ethical surveillance practices including consent requirements, transparent policies, and limited data retention periods.
Privacy impact assessments evaluate potential risks before deployment.
Public oversight committees provide accountability, while regulatory compliance guarantees adherence to privacy laws.
These safeguards aim to balance legitimate security needs with fundamental privacy rights in increasingly monitored environments.
What’s the Accuracy Difference Between Edge and Cloud-Based Detection Solutions?
Cloud-based solutions typically achieve 5-10% higher accuracy metrics due to greater computational resources, but edge solutions are catching up fast.
Smart deployment strategies can minimize this gap! Cloud excels with complex scenes requiring deep analysis, while edge systems like Detectum deliver 85-95% accuracy with dramatically lower latency.
Remember, the “best” solution depends on your specific needs—sometimes that real-time response trumps a marginal accuracy boost.
Don’t chase perfection when “good enough, right now” wins the day.
How Frequently Must Object Detection Models Be Retrained?
Object detection models typically require retraining every 3-6 months, but this varies widely.
Watch for data drift—when new objects appear or lighting conditions change, accuracy plummets fast! Model performance monitoring is your best friend here.
Environmental changes demand more frequent updates, while stable settings might stretch to yearly retraining.
The golden rule? Don’t wait for catastrophic failure. Monitor, test, and retrain when performance metrics start sliding, not after they’ve crashed completely.