AI is revolutionizing engineering across industries, not just making things faster but fundamentally smarter. It analyzes massive datasets to spot patterns humans miss, optimizes electricity generation, improves aircraft designs, and monitors infrastructure health before catastrophic failures occur. Predictive maintenance systems keep manufacturing equipment running while generative design algorithms create components that are simultaneously lighter and stronger. Engineers aren’t being replaced—they’re being supercharged with tools that transform impossible challenges into tomorrow’s innovations.
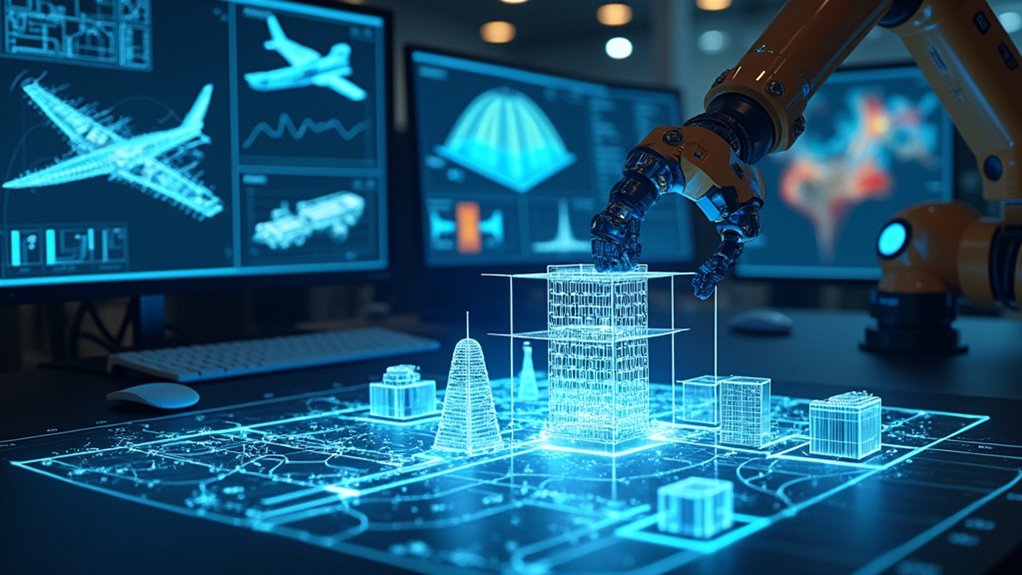
Artificial intelligence is revolutionizing engineering across industries, transforming how professionals design, build, and maintain our world. Traditional engineering challenges that once required extensive manual calculations and trial-and-error approaches are now being tackled through sophisticated AI applications.
These smart systems parse massive datasets in seconds, identifying patterns human engineers might miss. Don’t think this is just about replacing human ingenuity—it’s about amplifying it. Engineers now deploy machine learning algorithms to optimize electricity generation, preventing outages before they happen and making our power grid more reliable.
AI doesn’t replace engineers—it supercharges their capabilities, turning data into preemptive solutions humans might otherwise miss.
Ever wonder why your smartphone battery lasts longer than last year’s model? Thank AI-driven materials science innovations.
In aerospace engineering, AI systems analyze flight test data to refine aircraft designs, boosting fuel efficiency and cutting emissions. These algorithms simulate countless flight scenarios without burning a drop of jet fuel.
Meanwhile, automotive engineers leverage AI to perfect vehicle aerodynamics and develop self-driving technologies that react faster than human drivers. Gone are the days of building dozens of physical prototypes—AI simulations test thousands of virtual designs overnight. Deep learning algorithms are continuously improving autonomous vehicle capabilities by analyzing sensor data.
Civil engineers now monitor bridge and building health with AI that detects microscopic structural changes, predicting potential failures before catastrophic collapse. The system doesn’t just sound alarms; it recommends specific maintenance actions.
In mechanical engineering, generative design algorithms produce components that are lighter, stronger, and use less material than traditionally designed parts. These AI tools don’t just suggest improvements—they create entirely new design approaches humans might never consider.
Across manufacturing sectors, predictive maintenance systems analyze equipment vibrations, temperatures, and performance metrics to spot problems weeks before failure. This slashes downtime and extends machine life. Quality control automation has dramatically improved product defect detection beyond what human inspectors can achieve. With 86% of engineers considering AI an important emerging technology, adoption continues to accelerate across the profession.
Frequently Asked Questions
What Qualifications Do Engineers Need to Work With AI Systems?
Engineers working with AI systems need a robust mix of qualifications.
Engineering degrees in computer science, data science, or related fields form the foundation. Technical certifications in platforms like TensorFlow or PyTorch validate specialized AI skills.
But don’t get comfortable—continuous learning is non-negotiable in this rapidly evolving field. The most successful engineers combine theoretical knowledge with hands-on experience, staying current through workshops and professional development.
How Does AI Impact Job Security for Traditional Engineering Roles?
AI threatens traditional engineering roles requiring repetitive tasks, with 20-40% of jobs potentially automated in the next decade.
Job evolution demands engineers adapt or face obsolescence—sorry, but that’s reality.
Skill adaptation is non-negotiable; upskilling in AI development, data analysis, and creative problem-solving becomes essential.
While routine positions disappear, new hybrid opportunities emerge for those who embrace change.
The message? Evolve your skillset, or watch your career prospects evaporate.
What Are the Ethical Concerns When Implementing AI in Engineering?
Implementing AI in engineering raises critical ethical concerns.
Bias mitigation remains challenging, as algorithms may perpetuate existing prejudices in design decisions. Data privacy becomes precarious when AI systems process sensitive engineering specifications.
Accountability frameworks often lag behind technological implementation, creating responsibility gaps when systems fail. Transparency requirements face resistance from companies protecting proprietary algorithms.
These issues aren’t just theoretical—they affect real projects, real infrastructure, and ultimately, real people. Engineers must confront these challenges head-on.
How Much Does Implementing AI in Engineering Typically Cost?
AI implementation costs in engineering vary dramatically, from $20,000 for basic solutions to over $500,000 for custom builds.
Budget considerations should account for your industry—healthcare and finance projects cost 30-50% more due to regulations, while manufacturing requires hefty hardware integration.
Small businesses, don’t panic! Monthly subscription options ($99-$1,500) exist.
The brutal truth? Your data quality will make or break your budget, regardless of how deep your pockets are.
Can AI Engineering Solutions Work Without Internet Connectivity?
Yes, AI engineering solutions can absolutely work without internet connectivity.
Many systems leverage offline capabilities to process data locally on devices or edge computing systems. Engineers can deploy pre-trained models that operate independently—perfect for remote construction sites, manufacturing facilities with spotty connections, or autonomous vehicles that can’t wait for the cloud to make decisions.
These offline AI solutions trade some real-time learning capabilities for reliability and data security—a worthwhile exchange in many industrial applications.