AI primarily relies on four processor types: GPUs for parallel processing, TPUs for low-latency applications, ASICs for energy-efficient specialized tasks, and neuromorphic chips that mimic human brain function. NVIDIA dominates the GPU market while Google leads in TPU innovation. Modern devices often include dedicated Neural Processing Units (NPUs) that handle AI workloads while conserving battery life. Your smartphone might already contain an AI chip you never knew about. The processor battle continues to revolutionize what’s possible in artificial intelligence.
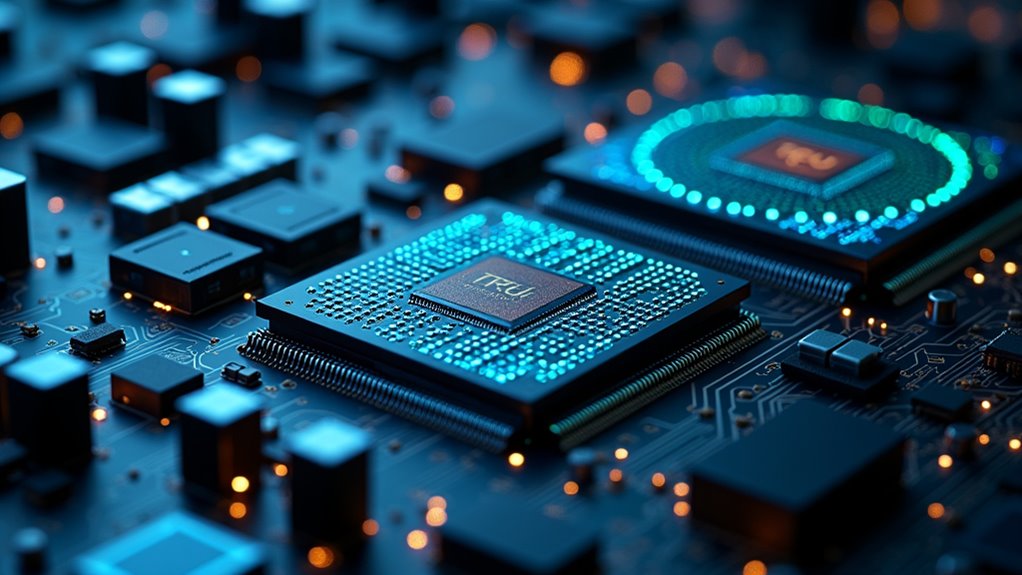
The beating heart of artificial intelligence, AI processors have transformed from specialized components to essential drivers of technological innovation. These processors aren’t your average computer chips—they’re specifically designed to handle the massive computational demands of AI algorithms.
AI hardware comes in various forms, each offering different levels of processor efficiency for specific applications. The right processor can mean the difference between an AI system that crawls along like a snail and one that processes information at lightning speed.
Choosing the right AI processor isn’t just a technical decision—it’s the difference between computational crawl and computational flight.
Several types of processors power today’s AI systems. GPUs (Graphics Processing Units) excel at parallel processing, making them ideal for handling multiple calculations simultaneously—something your standard CPU just can’t match efficiently.
When milliseconds matter, such as in autonomous vehicles, specialized processors like TPUs (Tensor Processing Units) developed by Google deliver the low latency required. For companies needing customized solutions, ASICs (Application-Specific Integrated Circuits) offer tailored performance with better energy efficiency.
Want AI that thinks more like a human brain? Neuromorphic chips are trying to mimic neural networks’ structure, potentially revolutionizing how machines learn. Meanwhile, quantum computing looms on the horizon, promising computational power that could solve problems current processors can’t even touch.
The big players aren’t sitting idle. NVIDIA dominates the GPU space, while Google pushes boundaries with their TPUs. Intel and AMD compete fiercely in the AI processor market, and even Apple has joined the game with AI-optimized chips in their devices.
These processors enable everything from the machine learning systems recognizing your face to the natural language processing powering your virtual assistant. They’re behind computer vision technologies in security systems and the brains of autonomous vehicles.
The processor you never see is silently powering the AI revolution all around you—from your smartphone to the cloud and everywhere in between.
Modern laptops now feature dedicated Neural Processing Units that significantly improve AI task efficiency while extending battery life during intensive workloads.
Frequently Asked Questions
How Much Power Do AI Processors Consume?
AI processors are power-hungry beasts. High-end GPUs like NVIDIA’s H100 devour up to 700W, while the newer Blackwell chips can gulp down a staggering 1,200 watts.
Energy consumption varies dramatically between training (power-intensive) and inference (more modest). Data centers housing these chips often require dedicated power plants just to keep them running!
The industry is scrambling for power efficiency solutions as AI’s electricity appetite approaches that of entire countries.
Can AI Processors Be Used for Non-Ai Applications?
Yes, AI processors can absolutely handle non-AI applications.
GPUs, originally designed for graphics, excel at parallel computing tasks like scientific simulations and data processing.
FPGAs offer remarkable versatility through reconfigurable hardware for various specialized tasks.
Even ASICs and TPUs, though more specialized, can tackle non-AI workloads requiring similar computational patterns.
The key to their non-AI compatibility lies in their parallel processing capabilities, which benefit applications beyond AI that need to crunch massive data simultaneously.
How Much Do Ai-Specific Processors Typically Cost?
AI-specific processors vary dramatically in cost. A price comparison reveals high-end chips from Nvidia fetching $30,000-$50,000, while AMD’s offerings hover around $10,000-$15,000.
Looking for budget options? Consumer-grade GPUs start around $2,000. The price tag depends on performance requirements, memory capabilities, and customization needs.
Manufacturing costs and market demand also influence pricing. Don’t expect these computational powerhouses to come cheap—but economies of scale might eventually make AI chips more affordable.
Are AI Processors Harmful to the Environment?
AI processors definitely harm the environment. Their production involves complex fabrication and resource extraction, leaving a substantial carbon footprint.
These power-hungry chips demand massive electricity for operation and cooling systems that guzzle water. The environmental impact extends beyond energy—think e-waste and mining damage.
While the tech industry is pushing toward sustainable technology through efficiency improvements and renewable energy integration, AI’s ecological cost remains significant.
Want greener AI? Look for companies prioritizing sustainability in their hardware choices.
Can AI Processors Be Upgraded in Existing Systems?
AI processors can be upgraded in existing systems, but processor compatibility is a major hurdle. Some devices offer interchangeable modules, while others are completely locked down—tough luck if you bought the latter!
Upgrade limitations include physical constraints (no, you can’t cram that new chip into your tiny smartwatch), cooling requirements, and cost considerations.
Cloud integration offers a workaround, letting users access beefier AI processing without replacing hardware. Software optimizations can also boost performance without physical upgrades.