AI essentials encompass narrow applications in daily life and powerful machine learning technologies. Companies leveraging AI gain competitive edges through automation and enhanced decision-making. Key concepts include pattern recognition, natural language processing, and generative capabilities—none of which constitute actual “understanding.” Ethical considerations remain critical, as AI systems often perpetuate biases from training data. Developing digital literacy helps navigate this landscape where algorithms increasingly influence our experiences. The technological roadmap ahead offers both remarkable potential and concerning limitations.
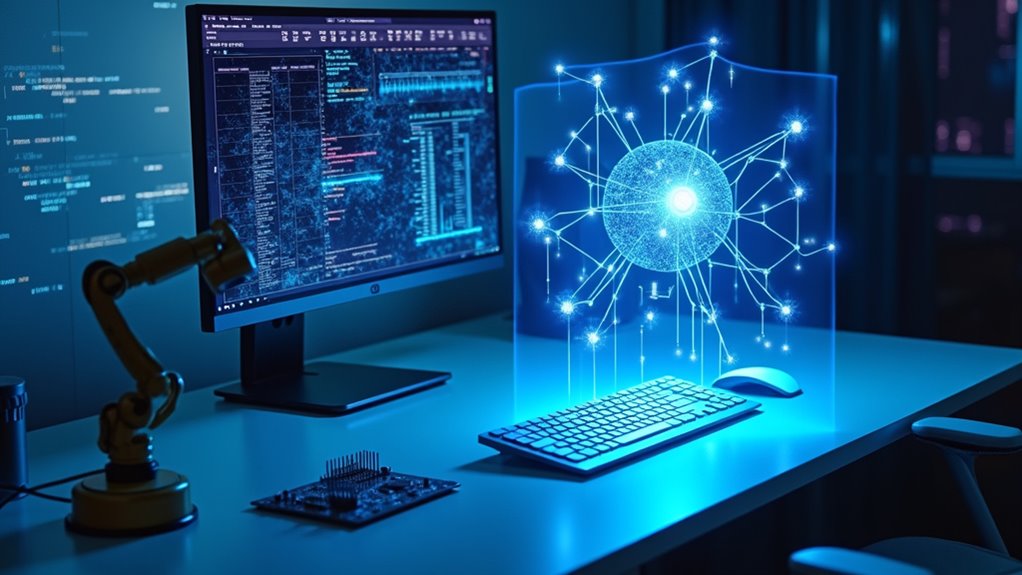
As our world becomes increasingly digitized, understanding the basics of artificial intelligence has never been more essential. AI applications now permeate countless aspects of daily life, from the smartphones in our pockets to the algorithms determining what content we see online.
Machine learning, a fundamental subset of AI, powers these systems by enabling computers to learn from data without explicit programming. You’re using AI right now, whether you realize it or not!
AI surrounds us invisibly, with machines quietly learning from data to shape our digital experiences.
Natural language processing lets machines understand and generate human language – that’s how virtual assistants recognize your commands and chatbots pretend to understand your complaints. These systems aren’t magic; they’re mathematical models trained on massive text datasets. Despite impressive language capabilities, current AI systems operate through pattern recognition techniques rather than genuine comprehension.
Generative models have dramatically evolved, creating eerily realistic images, videos, and text that can fool even discerning eyes. DALL-E, for instance, transforms text prompts into images that would have seemed impossible just years ago.
Ethical considerations must guide AI development. The same systems that offer convenience can perpetuate bias, invade privacy, and make decisions with real-world consequences. Microsoft has established core principles emphasizing responsible AI development that focuses on fairness, reliability, safety, and inclusivity.
Think your AI tools are neutral? Think again! Training data often contains societal biases that get baked right into the algorithms.
The business impact of AI continues to reshape industries through automation, personalization, and enhanced decision-making. Companies adopting AI judiciously gain competitive advantages, while those ignoring it risk obsolescence.
From predictive analytics to customer service chatbots, AI tools deliver measurable ROI when implemented thoughtfully.
AI categories range from narrow systems designed for specific tasks to the still-theoretical general AI with human-like capabilities. Understanding these distinctions helps set realistic expectations.
Deep learning, a subset of machine learning using neural networks, excels at finding patterns in complex datasets but requires substantial computational resources. Creating effective deep learning models often requires data analysis to identify trends and insights that inform the training process.
Want to navigate this AI-driven future successfully? Start by developing digital literacy, demanding transparency from AI systems you use, and recognizing both the potential and limitations of artificial intelligence.
Frequently Asked Questions
How Does AI Impact Job Security Across Different Industries?
AI’s impact on job security varies dramatically across sectors.
Manufacturing faces severe job displacement, with 2 million positions potentially gone by 2025.
Meanwhile, healthcare actually sees job creation for AI specialists.
Industry transformation hits hardest in routine task areas, while creative fields face new threats from generative AI.
Think your job is safe? Think again—14% of workers have already been displaced.
Even white-collar professionals aren’t immune, with 24% fearing obsolescence.
Adaptation is non-negotiable.
What Ethical Concerns Surround Ai-Powered Surveillance Systems?
AI-powered surveillance systems raise serious ethical red flags.
Privacy implications? Massive – these systems track your every move without consent.
Bias issues persist when algorithms unfairly target certain demographics, creating a digital discrimination nightmare.
Systems that can’t recognize diverse faces often misidentify minorities – oops, not so “intelligent” after all!
Plus, who’s watching the watchers? Without transparent oversight, surveillance tech becomes Big Brother’s dream and civil liberties’ worst nightmare.
Can AI Truly Develop Consciousness or Self-Awareness?
The consciousness debate in AI remains unresolved. Current systems merely simulate understanding without experiencing it.
Self-awareness implications are profound—if AI ever crossed this threshold, we’d face unprecedented ethical questions about machine rights. No, your chatbot isn’t secretly plotting world domination!
Despite impressive capabilities, today’s AI lacks the subjective experience that defines consciousness. The gap between sophisticated pattern recognition and true self-awareness remains vast—perhaps unbridgeable with current technology.
How Is AI Regulated Differently Across International Jurisdictions?
AI regulations vary dramatically across international jurisdictions, creating a patchwork of standards that tech companies must navigate.
While the EU embraces strict oversight with its extensive AI Act, the US favors a more hands-off, innovation-first approach.
Jurisdictional differences create compliance challenges for global organizations, who must simultaneously satisfy China’s state-security focus, Canada’s ethical frameworks, and Japan’s shifting governance models.
International standards remain elusive, with sovereignty concerns often trumping calls for global regulatory harmony.
What Computational Resources Are Required for Training Advanced AI Models?
Training advanced AI models demands significant computational muscle. High-end GPUs form the backbone, while cloud computing enables scalability without breaking the bank.
Massive data storage solutions are non-negotiable—you’ll need petabytes, not terabytes! Model optimization techniques reduce training time, but energy consumption remains staggering.
Hardware advancements continually push boundaries, though scalability challenges persist as models grow. The resource equation is simple: more parameters equal more hardware, more electricity, and more patience during those weeks-long training runs.