AI-powered image identification revolutionizes detection processes across industries. Using convolutional neural networks, these systems recognize patterns in images with remarkable accuracy—sometimes hitting 98% confidence levels. They’re not just spotting cats anymore; they’re driving cars, diagnosing diseases, catching manufacturing defects, and enhancing security systems. Real-world challenges like poor lighting or partial occlusion still exist, but continuous learning keeps improving performance. Edge computing solutions are making these processes even faster and more reliable. The tech’s future capabilities might surprise you.
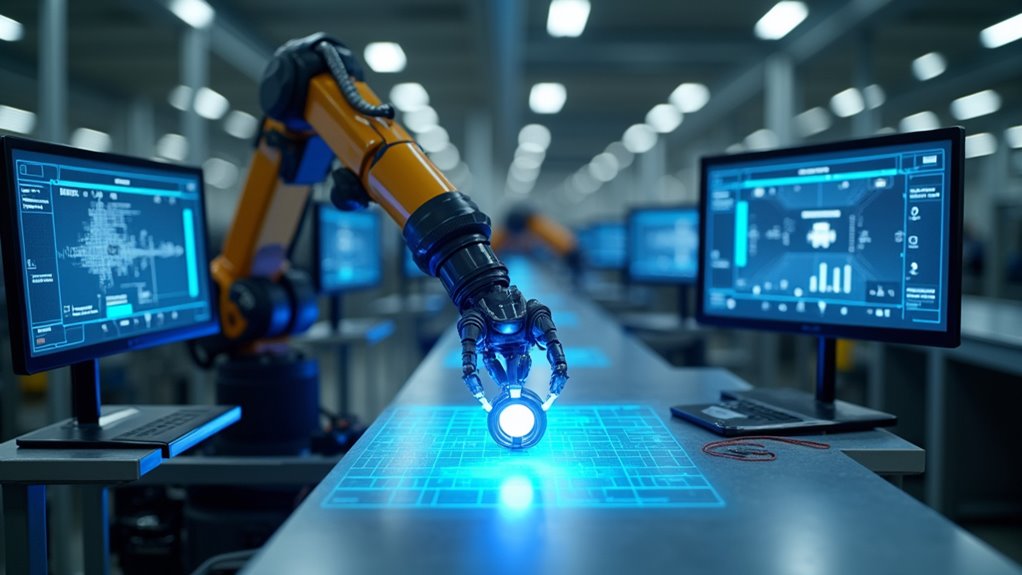
While humans can glance at a picture and instantly recognize a face, a dog, or a stop sign, computers need specialized training to perform the same feat. This training forms the backbone of AI image recognition, a revolutionary computer vision technique that’s transforming how machines interpret our visual world.
Modern image classification techniques have evolved dramatically, enabling everything from basic binary classifiers to sophisticated multiclass systems that can identify countless objects simultaneously. Don’t underestimate what these systems can accomplish—they’re not just identifying cats on the internet (though they excel at that too).
Real-time processing has become the gold standard for practical applications. Ever wonder how your phone recognizes faces so quickly? That’s AI working at lightning speed! These systems utilize Convolutional Neural Networks (CNNs)—essentially digital brains dedicated to pattern recognition.
CNNs work through encoder layers that learn to detect edges, shapes, and eventually complex objects like human faces or traffic signs. The fully connected layers then deliver those confidence scores that determine whether something is, say, 98% likely to be a stop sign.
These technologies power innovations across industries. Self-driving cars rely on image recognition to navigate safely. Hospitals use similar systems to spot anomalies in medical images that might escape even trained radiologists. Similar to KnowVID-19’s approach, these technologies utilize keyword-based classification to organize and categorize visual information effectively.
Manufacturing facilities deploy these tools for quality control, catching defects that would slip past human inspectors. The accuracy of these systems depends heavily on training data—garbage in, garbage out, as they say. Emerging edge computing solutions are enabling more efficient real-time processing directly on devices without requiring constant cloud connectivity.
Models trained on ImageNet and similar massive datasets typically perform best, but even they struggle with real-world variations. Lighting changes, unusual angles, partial occlusions—all can confuse even sophisticated AI systems. The technology achieves greater accuracy through continuous learning from vast amounts of stored image data.
Despite these challenges, AI-powered image identification continues to advance. The technology that once struggled to tell cats from dogs now performs complex facial recognition through security cameras, counts inventory on store shelves, and helps doctors diagnose diseases.
The future of visual processing isn’t just bright—it’s crystal clear.
Frequently Asked Questions
How Secure Is AI Image Identification Against Adversarial Attacks?
Current AI image identification systems remain vulnerable to adversarial attacks, with detection accuracy often compromised by deliberately crafted manipulations.
Despite progress in adversarial robustness techniques, most systems can be fooled through frequency domain tampering or pixel-level modifications.
Researchers continue developing defensive approaches like adversarial training and Bayesian strategies, but the cat-and-mouse game persists.
Bottom line? These systems aren’t nearly as secure as their creators might want you to believe.
Can AI Image Systems Identify Objects in Extreme Weather Conditions?
AI image systems can identify objects in extreme weather conditions, but face significant weather challenges.
Rain, fog, and snow often degrade image quality, making object recognition trickier than on sunny days. Advanced models compensate by training on diverse weather datasets and using multiple sensors (radar, infrared) to “see” through harsh conditions.
Still, performance typically drops by 15-30% in extreme weather compared to ideal conditions. The industry’s working on it, but Mother Nature remains a formidable opponent!
What’s the Carbon Footprint of Running AI Image Identification Systems?
The carbon footprint of AI image identification systems varies widely.
Daily operations generate relatively modest carbon emissions compared to the massive energy consumption required during training phases. Running a single image through identification systems produces emissions equivalent to driving a car for a few feet—seems tiny, right?
But multiply that by millions of daily uses, and you’ve got a significant environmental impact. Location matters too; systems powered by renewable energy create substantially lower footprints than those using coal-based electricity.
How Often Should AI Image Identification Models Be Retrained?
Retraining AI image identification models depends on model performance and data freshness. No one-size-fits-all schedule exists.
Most organizations monitor performance metrics and retrain when accuracy drops below acceptable thresholds. Data drift happens, folks—sometimes weekly in rapidly changing environments, monthly in moderate ones, or quarterly in stable scenarios.
The golden rule? Don’t wait for model collapse. Regular evaluation beats emergency retraining every time.
Are There Legal Implications When Misidentifications Occur in Critical Applications?
Misidentifications in critical applications carry serious legal consequences.
When AI systems fail to identify correctly in healthcare, security, or transportation, misidentification liability can result in lawsuits, financial damages, and regulatory penalties.
Who’s responsible? Developers, users, or the AI itself? The legal landscape remains murky.
Ethical considerations demand transparent error reporting and appropriate safeguards.
Companies must implement robust testing protocols and human oversight—because when AI gets it wrong in life-or-death situations, the consequences aren’t just technical, they’re tragically human.