AI image detection has become essential as fake content floods our digital world. Look for telltale signs: weird fingers (six, anyone?), too-perfect hair, unnatural blurring, or oddly neglected backgrounds. Current tools aren’t foolproof—CNN and YOLO algorithms help, but they’re racing to keep up with advancing AI generators. Train your skeptical eye by practicing manual evaluation techniques like examining fine details. The battle between detection technology and generation capabilities is just heating up.
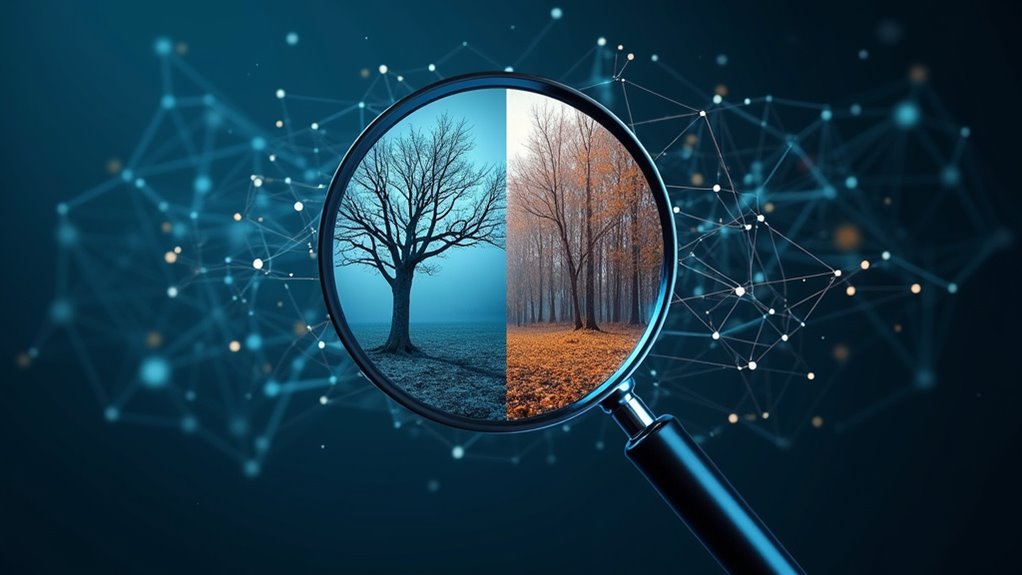
As artificial intelligence continues to reshape our visual landscape, the ability to detect AI-generated images has become increasingly essential. The proliferation of sophisticated machine learning tools has created a digital environment where content authenticity is no longer guaranteed. Can you tell what’s real anymore? Probably not—and that’s exactly the problem.
The technology behind AI image detection relies heavily on Convolutional Neural Networks (CNNs), which excel at recognizing patterns in visual data. YOLO (You Only Look Once) algorithms provide real-time detection with impressive accuracy, while more complex systems like Mask R-CNN add the ability to outline objects with precision. Deep learning methods offer superior performance and flexibility compared to traditional computer vision techniques for these detection tasks.
CNN technology forms the backbone of AI detection, with YOLO algorithms delivering speed while Mask R-CNN brings precision to the digital authenticity battle.
These aren’t just fancy tech terms—they’re the digital bloodhounds sniffing out what’s real and what’s fake.
Looking to spot AI images yourself? Check for those telltale visual errors. Count the fingers in that suspiciously perfect hand (spoiler: there might be six). Examine hair and eyes—AI often renders these with unnatural uniformity. Strange blurring or warping? Classic AI giveaway. The background often holds clues too, as AI tends to focus on the main subject while neglecting details elsewhere.
Detection tools are improving, but they’re far from perfect. They’ll flag an AI image one day and miss it the next. Companies are experimenting with invisible watermarking for AI content, but we’re not there yet. The SIFT method can be a valuable approach when manually evaluating suspicious content. Until then, your best defense is practice and skepticism.
The challenges aren’t just technical—they’re ethical and legal too. As AI-generated content becomes indistinguishable from human work, we need new frameworks for attribution and transparency. The value of creativity itself is at stake.
Remember: computational efficiency remains a significant hurdle in detection efforts. Even the most advanced algorithms struggle with the balance between accuracy and processing speed.
Popular platforms like Google Cloud Vision and Amazon Rekognition are integrating AI detection capabilities into their broader image analysis services, creating more accessible tools for professionals and consumers alike.
In this cat-and-mouse game between creation and detection technologies, staying informed is your best strategy. Trust nothing, verify everything.
Frequently Asked Questions
Can AI Detection Tools Identify Specific Generative Models Used?
Some AI detection tools can identify specific generative models, but model identification capabilities vary widely.
Tools like Illuminarty analyze pixel patterns to determine which AI created an image, while simpler options like BrandWell just flag content as AI-generated without specifying the source.
Detection accuracy remains imperfect, with frequent false positives.
The field’s evolving rapidly, though—don’t expect perfection! As generative AI advances, detection tools must constantly update to recognize new models’ unique fingerprints.
How Often Do AI Detection Tools Release Updates?
AI detection tools typically release major updates quarterly or bi-annually, with minor fixes appearing monthly.
Update frequency directly impacts tool reliability, as these systems must evolve to catch increasingly sophisticated AI generators. Companies rush to keep pace with advancements in models like DALL-E and Stable Diffusion.
Want dependable detection? Look for tools that transparently communicate their update schedule—those updated less than quarterly are likely falling behind in the endless cat-and-mouse game.
Do AI Detectors Work on Edited or Compressed Images?
AI detectors struggle greatly with edited or compressed images.
Image compression strips away pixel data that detectors rely on, often leading to false results.
Similarly, editing techniques can mask telltale AI artifacts.
Want accurate detection? Avoid heavy compression and minimal editing.
The reality is uncomfortable but clear: as compression increases and editing gets more sophisticated, detection accuracy plummets.
This creates a frustrating cat-and-mouse game between detection tools and those trying to disguise AI origins.
Can Ai-Generated Images Bypass Detection Through Simple Modifications?
Yes, AI-generated images can indeed bypass detection through simple modifications.
Basic image manipulation techniques—like adding noise, applying filters, or subtle compression—regularly confuse detection algorithms. These adjustments exploit fundamental detection challenges, making even sophisticated systems struggle to distinguish real from fake.
What’s concerning? The most effective evasion techniques often require minimal technical skill.
Adversarial attacks specifically designed to fool detectors are becoming increasingly accessible, raising serious questions about the reliability of current detection methods.
Are There International Standards for AI Image Detection Capabilities?
International standards for AI image detection are emerging but still developing. Organizations like ISO/IEC committees and the Partnership on AI are working to establish universal guidelines, while technical frameworks like C2PA and IPTC promote standardized watermarking and metadata solutions.
These efforts aim to improve detection accuracy across platforms, though they face challenges from adversarial tactics. Unlike your kitchen junk drawer, these standards can’t be a messy free-for-all—too much is at stake for global information integrity.