AI-powered data analytics is revolutionizing how businesses extract insights from information. Companies now enjoy up to 25% cost savings through automated systems that clean data and predict trends without human error. These tools spot patterns humans miss, visualize complex relationships in intuitive dashboards, and transform customer comments into actionable strategies—all before your morning coffee. No advanced degree needed to interpret the results. Businesses must adapt to this real-time decision-making landscape or watch competitors zoom ahead.
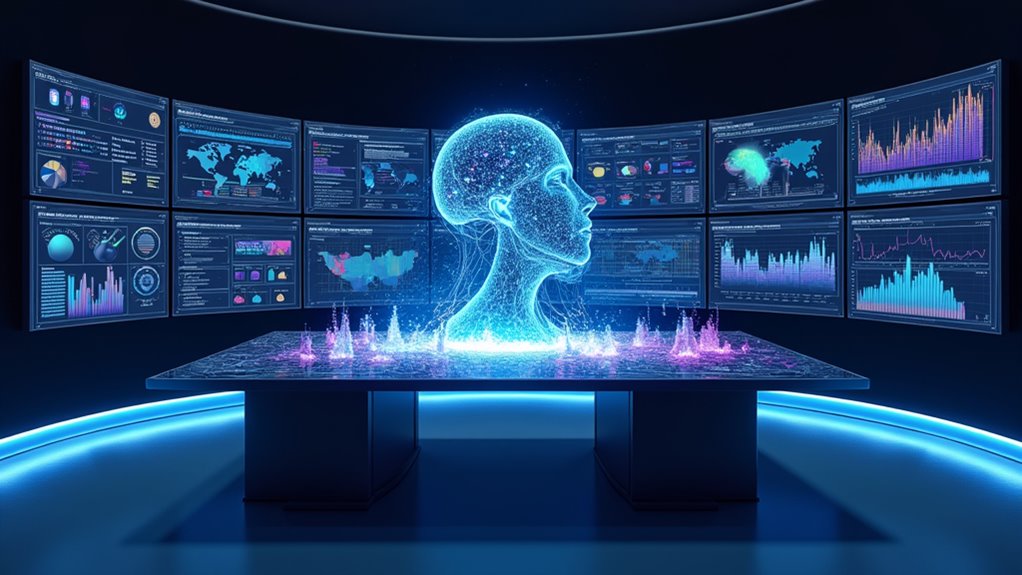
While businesses have always relied on data to drive decisions, the emergence of AI-powered analytics has revolutionized how companies extract value from their information assets. Gone are the days of spreadsheet gymnastics and manual number-crunching. Today’s data automation systems pull information from multiple sources simultaneously, clean it up, and serve insights on a silver platter—all before your morning coffee gets cold.
The magic behind this transformation? Predictive modeling algorithms that don’t just tell you what happened yesterday but forecast what might happen tomorrow.
Organizations implementing AI analytics are seeing impressive returns—up to 25% cost savings through streamlined operations. Let’s be honest: humans make mistakes, especially when drowning in spreadsheet cells at 5 PM on a Friday. AI doesn’t get tired, doesn’t daydream about weekend plans, and certainly doesn’t accidentally delete vital formulas. The result? More reliable insights without the human error baggage.
AI never needs coffee breaks or makes Friday afternoon errors—just consistent insights that save businesses up to 25%.
AI’s pattern detection capabilities are downright spooky. It spots anomalies in massive datasets that would take human analysts weeks to find—if they found them at all. These tools aren’t just fancy tech toys; they’re competitive necessities in industries from healthcare to retail. AI’s ability to provide predictive analytics capabilities helps organizations forecast trends based on historical data for more informed strategic planning. These systems excel at anomaly detection by identifying deviations from normal operational patterns, enabling timely interventions before problems escalate. Imagine knowing a machine will fail before it breaks down or predicting customer behavior before your competitors even notice the trend.
Natural Language Processing turns messy customer comments into actionable insights, while visualization tools transform complex data relationships into intuitive dashboards anyone can understand. No PhD required.
The integration challenges aren’t trivial, though. Remember: AI systems are only as good as the data they consume. Feed them garbage, and you’ll get garbage predictions—just faster and with more confidence than before.
For businesses still relying on traditional analytics methods, the message is clear: adapt or be left behind. When your competitors are making decisions in real-time based on AI-powered insights, last quarter’s reports won’t cut it anymore.
The future of business intelligence isn’t just about having data—it’s about having AI that makes that data truly intelligent. Effective implementation requires intelligent oversight to ensure AI solutions enhance human capabilities rather than simply replacing them.
Frequently Asked Questions
How Much Technical Expertise Is Needed to Implement AI Analytics?
Implementing AI analytics requires varying levels of technical skills depending on role complexity.
Data scientists need deep expertise in machine learning and programming, while business users can leverage user-friendly platforms with minimal coding knowledge.
Successful implementation strategies blend technical prowess with domain expertise. Organizations should focus on building cross-functional teams where technical specialists collaborate with subject matter experts.
What’s the ROI Timeline for AI Analytics Investments?
ROI timelines for AI analytics investments vary wildly. Most companies see returns within 1-3 years, though some experience faster results—as quick as 12 months.
Don’t get starry-eyed! ROI benchmarks depend on your data quality (garbage in, garbage out) and deployment complexity.
Smart investment strategies include starting with targeted projects, continuously monitoring performance, and managing expectations.
How Does AI Analytics Handle Data Privacy Concerns?
AI analytics addresses privacy concerns through multiple approaches. Organizations implement data encryption to protect sensitive information from unauthorized access.
User consent mechanisms guarantee legal compliance and build trust. Privacy-preserving techniques like federated learning allow analysis without exposing raw data.
Robust governance frameworks help companies navigate regulatory requirements. However, challenges remain—the balance between insight extraction and privacy protection requires ongoing vigilance, and no solution is foolproof without proper implementation and oversight.
Can AI Analytics Integrate With Existing Legacy Systems?
Yes, AI analytics can integrate with legacy systems, but compatibility isn’t always straightforward.
Companies face integration challenges when connecting modern AI tools to outdated infrastructure. Solutions include APIs, middleware, and data lakes that serve as bridges between old and new.
Smart organizations implement phased approaches—tackle one connection point at a time rather than attempting complete overhauls.
What Are the Common Pitfalls When Adopting AI Analytics?
Common AI analytics pitfalls include overlooking data quality issues—garbage in, garbage out is no joke!
Organizations often underestimate the importance of thorough data cleansing before implementation. Inadequate user training creates a painful adoption curve, leaving powerful tools gathering digital dust.
Other traps include unrealistic expectations about immediate ROI, insufficient infrastructure planning, and ignoring privacy regulations.