AI product recognition transforms retail by automatically identifying items through images. This tech uses fancy neural networks (don’t worry, they’re just math that mimics brains) to monitor inventory, power visual searches, and speed up checkout—all without those pesky barcodes. Sure, poor lighting and similar packaging can trip it up, but the efficiency gains are massive. Stop counting inventory manually like it’s 1995! Discover how this visual wizardry might make cashiers obsolete.
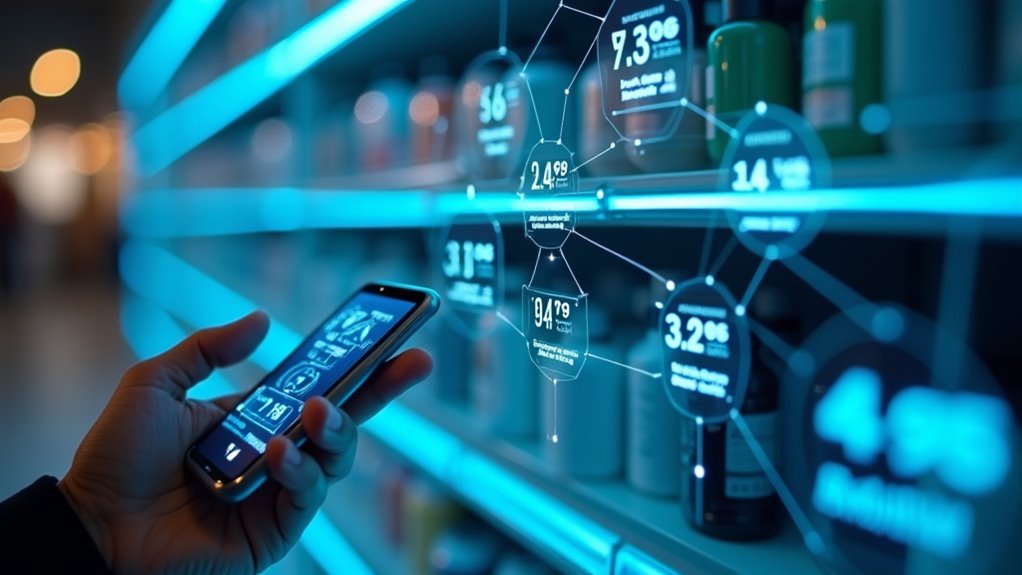
As retail technology continues to evolve at breakneck speed, AI product recognition has emerged as a game-changing innovation that’s transforming how businesses track inventory and serve customers. These sophisticated systems leverage image classification and machine learning algorithms to identify products automatically—no human squinting required. Retailers who ignore this tech might as well be counting inventory with an abacus while competitors zoom ahead.
AI product recognition isn’t optional anymore—it’s the difference between counting beans manually or running a modern, competitive retail business.
The technology isn’t just fancy gadgetry; it’s solving real-world problems across multiple domains. In retail management, these systems prevent those frustrating “sorry, we’re out of stock” moments by monitoring inventory levels in real-time. Visual product search capabilities let consumers find items by simply pointing their phones at similar products—much like Google Lens, but specifically tuned for shopping experiences. Users can easily test this functionality by visiting the Product Recognition site and submitting pictures of products for immediate identification. The integration of ethical AI principles can help mitigate the spread of health misinformation when these systems are applied to medical product recognition on social media platforms.
Behind the scenes, the technical machinery is impressive. Convolutional Neural Networks (CNNs) do the heavy lifting, analyzing thousands of visual features to distinguish between products. Think of them as extremely picky observers that can tell the difference between nearly identical cereal boxes in milliseconds. Frameworks like TensorFlow and PyTorch provide the foundation, while libraries such as OpenCV handle the nitty-gritty of image processing. These neural networks form the technical foundations of modern computer vision that powers everything from social media filters to autonomous driving systems.
Of course, it’s not all smooth sailing. Poor lighting, occluded products, and similar packaging designs can trip up even the best systems. Data quality issues remain a persistent challenge—garbage in, garbage out still applies, even with AI! And let’s not forget the computational resources needed; these systems are hungry beasts.
The business benefits, however, are undeniable. Operational efficiency skyrockets as manual inventory becomes automated. Checkout lines move faster when products are recognized instantly. And theft prevention improves dramatically when systems can spot when items mysteriously disappear from shelves.
As AI continues to advance, expect these systems to become even more accurate, faster, and integrated into the shopping experience—perhaps one day making the barcode as obsolete as the telegraph.
Frequently Asked Questions
How Much Does AI Product Recognition Technology Typically Cost?
AI product recognition costs vary dramatically based on several key cost factors.
Basic solutions start around $5,000, while extensive enterprise systems can exceed $500,000. Various pricing models exist – subscription services might charge monthly fees while custom solutions require upfront investment.
Hardware requirements, data acquisition, and AI expertise greatly impact the final price tag. Most businesses find middle-range solutions ($50,000-$150,000) offer the best balance between functionality and affordability.
Can AI Identify Counterfeit Products From Images?
Yes, AI can identify counterfeit products from images. Modern visual AI algorithms excel at counterfeit detection by analyzing subtle differences in packaging, logos, and product features that humans might miss.
These systems compare suspicious items against databases of authentic products, flagging anomalies for brand protection teams. However, the technology isn’t foolproof—sophisticated counterfeits can sometimes evade detection, and AI systems require constant updates to keep pace with increasingly clever fake product tactics.
What Privacy Concerns Exist With Automated Product Recognition?
Automated product recognition raises serious privacy concerns. Companies often collect data without proper consumer consent, scanning photos that may contain personal items or environments.
Data security remains woefully inadequate in many systems, with information vulnerable to breaches. Plus, who’s tracking what happens to your shopping habits after they’re recorded?
The technology can create detailed consumer profiles without shoppers realizing it. Remember, that convenient feature identifying your perfume might be remembering more than you bargained for.
How Long Does Implementing a Product Recognition System Take?
Implementation timelines for product recognition systems vary dramatically. Simple systems can take 1-2 months, while complex ones might stretch to 6+ months.
The project management approach matters too! Data quality (garbage in, garbage out, folks), team expertise, and technology stack all impact your timeline.
Remember, rushing AI implementation is like skipping breakfast before a marathon—technically possible but painfully unwise.
Most companies underestimate integration time, so add a buffer to whatever timeline you’re dreaming up.
What Error Rates Should Companies Expect With This Technology?
Companies should expect variable error rates with product recognition technology.
Industry accuracy benchmarks range from 5-20% error rates for basic implementations, but can drop below 5% in controlled environments.
Lighting, product complexity, and algorithm quality dramatically impact results.
Want better performance? Invest in quality data and top-tier algorithms.