Integrating AI into business strategy requires methodical planning, not just tech splurging. First, identify specific problems AI can solve—customer service headaches or predictive maintenance opportunities. Assess your data quality (garbage in = garbage out), then implement pilot projects to prove value before scaling. Choose technologies that match your objectives, whether that’s machine learning or natural language processing. Don’t forget the human element—your team needs training and buy-in. The path to AI success involves strategic patience and continuous refinement.
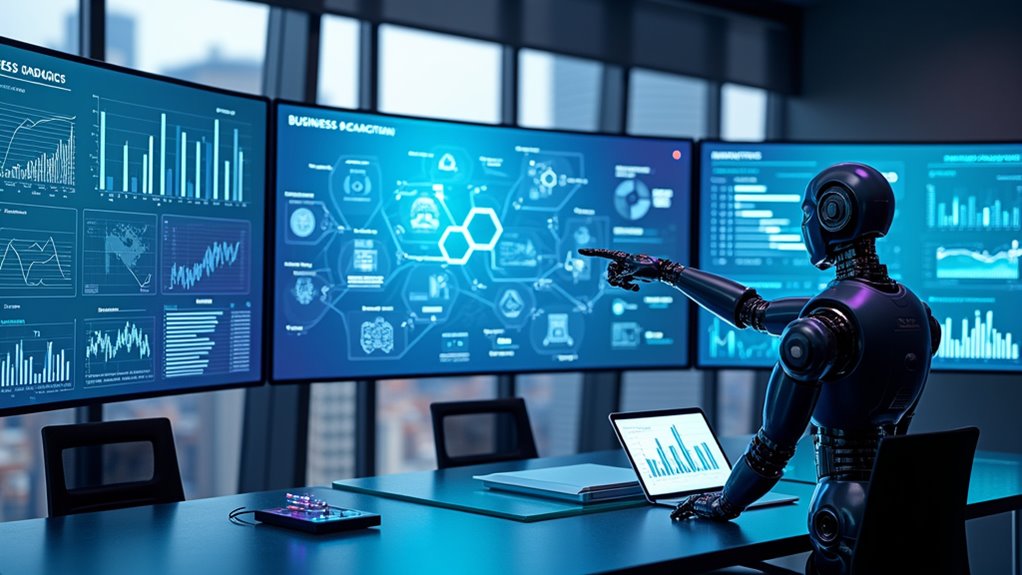
Why are industry leaders increasingly turning to artificial intelligence as their secret weapon? It’s simple—AI delivers competitive advantages that traditional approaches simply can’t match. Companies leveraging AI enjoy enhanced decision-making capabilities, operational efficiencies, and the ability to anticipate market shifts before competitors even notice them happening.
Successful AI Implementation begins with a thorough assessment of your current technological infrastructure. Don’t just jump on the AI bandwagon because it’s trendy—that’s a rookie mistake. Start by identifying specific business problems that AI could actually solve, then align these opportunities with your broader Strategic Alignment goals. Establishing clear ethical frameworks for AI use is essential to build trust with stakeholders and ensure responsible implementation. Consider integrating custom variables to enhance AI functionality and maintain greater control over content generation.
Start with business problems, not trendy AI solutions. True digital transformation demands strategy before technology.
Yes, this means you’ll need to evaluate your data quality first. Sorry, but garbage data produces garbage insights, no matter how sophisticated your algorithms.
The integration of AI requires a phased approach. Begin with pilot testing—a small, controlled environment where failure won’t sink your entire operation. Track results obsessively, measuring both quantitative metrics and qualitative feedback. Only after proving concept should you consider scaling across departments.
Selecting appropriate AI technologies demands careful consideration. Machine learning platforms offer broad capabilities, while natural language processing might better serve customer-facing operations. Choose tools that match your specific objectives, not just whatever your competitor is using. Implementing process automation solutions can significantly reduce manual workloads while improving accuracy and consistency across operations.
Organizations face legitimate challenges during AI adoption. Talent acquisition remains particularly difficult—good luck finding affordable AI specialists in today’s market! Infrastructure upgrades often require significant investment, and the cultural shift needed shouldn’t be underestimated. Employees must understand that AI augments their capabilities rather than threatens their jobs.
Remember that AI isn’t a set-it-and-forget-it solution. Continuous monitoring, refinement, and adaptation are essential as technologies evolve and business needs shift. Create feedback loops that inform ongoing improvements, and don’t be afraid to pivot when initial approaches underperform.
The most successful AI strategies remain flexible, data-driven, and focused on delivering measurable business value rather than chasing technological novelty.
Frequently Asked Questions
What Are the Ethical Implications of Implementing AI?
Implementing AI brings significant ethical challenges. Companies must address bias mitigation to prevent algorithms from amplifying societal prejudices.
Privacy concerns loom large as AI systems collect vast amounts of personal data. Organizations need robust accountability frameworks—who’s responsible when AI makes harmful decisions?
Transparency requirements are non-negotiable; “black box” algorithms undermine trust. These aren’t just theoretical concerns—they’re business imperatives.
Without ethical guardrails, AI implementation risks damaging brand reputation, triggering regulatory backlash, and eroding customer confidence.
How Much Does AI Implementation Typically Cost?
AI implementation costs vary dramatically based on scope and complexity.
Small businesses typically budget $5,000-$30,000 initially, while large corporations might spend $200,000 to millions.
Don’t forget ongoing expenses—they’ll eat up 35-95% of your initial investment annually!
Smart implementation strategies include starting with off-the-shelf solutions (as low as $0-$25,000/year) before diving into custom development.
Budget considerations should factor in data preparation, integration complexity, and maintenance engineering—those sneaky costs that everyone forgets about until it’s too late!
Will AI Replace Human Employees in My Business?
AI won’t entirely replace your workforce, but it will transform it.
Complete job replacement? That’s overblown.
What’s actually happening is job evolution, where AI handles repetitive tasks while humans focus on creativity and complex problem-solving.
Smart businesses are embracing AI collaboration—pairing human ingenuity with AI efficiency.
Your employees aren’t obsolete; they simply need new skills.
Want to stay competitive? Don’t replace workers—retrain them to work alongside AI.
The future isn’t man vs. machine, it’s man with machine.
What Security Risks Come With Adopting AI Solutions?
Adopting AI introduces serious security concerns.
Data privacy risks emerge when systems mishandle sensitive information—yep, your customer data could leak faster than office gossip.
Cyber threats multiply as attackers leverage AI to craft sophisticated phishing schemes that even your tech-savvy employees might fall for.
Adversarial attacks can trick AI systems into making dangerous decisions.
Companies must implement robust access controls, regular security audits, and thorough monitoring.
How Long Does AI Integration Usually Take?
AI integration isn’t a sprint—it’s a marathon. The implementation timeline typically spans 3-12 months, depending on project complexity.
Planning takes weeks to months, data preparation can drag on for months (garbage in, garbage out!), and model development adds several more weeks.
Integration challenges often include data quality issues, legacy system compatibility, and organizational resistance.