AI transforms IT data analysis by processing massive datasets in minutes instead of weeks. Machines consistently outperform humans in accuracy, spotting patterns and flagging anomalies without fatigue-induced errors. Instead of reacting to problems, AI anticipates challenges before they arise, providing real-time insights into system performance. It automates mundane tasks like data cleaning, freeing analysts to focus on strategic initiatives. The technology’s learning algorithms continuously improve, making today’s impressive capabilities just the beginning of what’s possible.
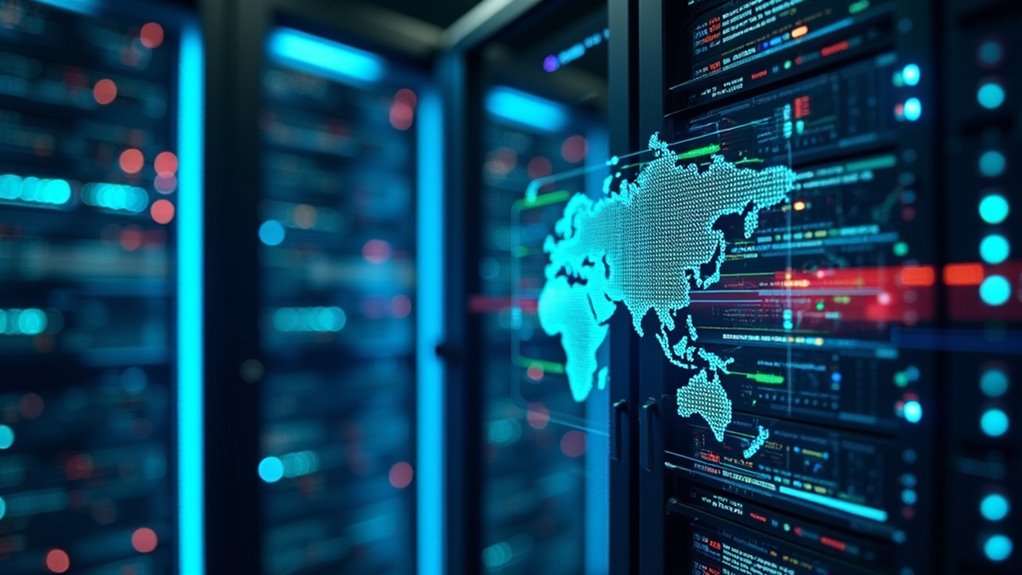
Revolutionizing the world of information technology, artificial intelligence has transformed how businesses analyze their IT data. Recent AI advancements have enabled organizations to process massive datasets in minutes rather than weeks, delivering insights at unprecedented speeds. The evolution of data integration capabilities means companies can now seamlessly combine structured and unstructured information from disparate sources—something that would’ve given your IT team migraines just a few years ago.
AI’s impact on error reduction cannot be overstated. Human analysts make mistakes; they get tired, distracted, or simply overwhelmed by data volume. Machines don’t. They process information with consistent accuracy, spotting patterns humans might miss and flagging anomalies that could indicate serious issues. This minimal error rate in AI data processing significantly enhances the overall accuracy of IT systems.
The relentless precision of AI outperforms human analysis, catching hidden patterns and critical anomalies human eyes inevitably miss.
The predictive capabilities of AI have fundamentally altered how businesses approach decision-making. Rather than simply reacting to problems, organizations can now anticipate challenges before they materialize. Think of it as having a crystal ball, but one powered by algorithms instead of mystical energy.
Real-time insights represent another game-changing benefit. When market conditions shift or system performance fluctuates, AI-powered analytics detect these changes instantly. No more waiting for monthly reports to discover what went wrong three weeks ago! AI analytics can effectively identify security anomalies by using computer vision techniques to analyze potential visual security breaches in IT infrastructure.
Machine learning algorithms continuously improve their accuracy by learning from new data, while natural language processing makes sense of text-based information. Deep learning, with its complex neural networks, tackles the most sophisticated analysis challenges, from image recognition to sentiment analysis. Organizations must carefully address ethical considerations surrounding privacy and bias when implementing these AI systems for data analytics.
Beyond technical benefits, AI liberates human analysts from mundane tasks. Data cleaning no longer consumes precious hours, freeing professionals to focus on strategic initiatives instead. This automation drives operational efficiency while enhancing collaboration through standardized insights accessible across departments.
From financial forecasting to fraud detection, AI’s applications span industries. Healthcare providers utilize AI to analyze medical images, retailers personalize shopping experiences, and manufacturers predict equipment failures before they happen. The difference between mere analysis and actionable analytics has never been clearer—or more valuable.
Frequently Asked Questions
What Security Risks Arise With AI in IT Data Analysis?
AI in IT data analysis introduces significant security risks.
Organizations face malicious attacks like data poisoning and model inversion, which can compromise critical systems.
Data privacy concerns loom large—AI might inadvertently expose sensitive information through prompt injection vulnerabilities or unsecured outputs.
Don’t forget the hardware vulnerabilities! Physical side-channel attacks can exploit processing weaknesses.
And here’s the kicker: overreliance on AI without human oversight creates blind spots that attackers love to exploit.
How Much Training Do IT Staff Need for AI Systems?
IT staff require multi-layered training for AI systems, starting with foundational AI literacy and progressing to role-specific skills.
Basic AI training requirements include understanding machine learning concepts, data analysis fundamentals, and ethical considerations—this isn’t optional after February 2025, thanks to the EU AI Act.
Staff skill development should follow a modular approach, with documentation of all training efforts.
The depth needed varies by role; frontline users need operational knowledge, while developers require more technical expertise.
Can AI Analyze Data Across Heterogeneous IT Environments?
Yes, AI excels at analyzing data across heterogeneous IT environments.
Through cross-platform integration, AI systems can connect disparate systems that previously couldn’t “talk” to each other. Data normalization techniques transform inconsistent formats into standardized structures—no more apples-to-oranges comparisons!
AI’s pattern recognition abilities work across platforms, identifying anomalies and trends human analysts might miss. The real magic happens when these systems learn over time, continuously improving their analysis capabilities across your messy, real-world IT landscape.
What’s the ROI Timeline for Implementing AI Data Analysis Tools?
ROI timelines for AI data analysis tools vary, but follow a predictable curve. Implementation strategy matters—most organizations see quick wins within weeks through task automation, with substantial returns appearing within 6-12 months.
Your ROI assessment should track both hard metrics (cost savings, productivity) and soft benefits (improved decision-making).
Setup can be surprisingly quick—some solutions deploy in under an hour. Remember, the industry average suggests $3.50 returned for every dollar invested in AI analysis tools.
How Do AI Systems Handle Incomplete or Corrupted IT Data?
AI systems tackle messy IT data through several clever approaches. First, data imputation fills those annoying gaps with educated guesses based on existing patterns—no more incomplete entries ruining everything!
Meanwhile, anomaly detection algorithms sniff out corrupted data like digital bloodhounds, flagging suspicious values before they poison your analysis. These systems don’t just give up when facing dirty data; they roll up their algorithmic sleeves and clean house, preserving analytical integrity where humans would waste hours manually fixing problems.