An AI model is fundamentally a digital brain that analyzes patterns in data to make predictions or decisions. These sophisticated algorithms use neural networks—layers of artificial neurons—to process complex information, learning from experience without coffee breaks. Unlike humans, they never tire. From spam filters to Netflix recommendations, AI models quietly influence your daily life. They’re trained on massive datasets, continuously tweaked for better performance. Understand these systems now; they’re already determining more of your future than you might realize.
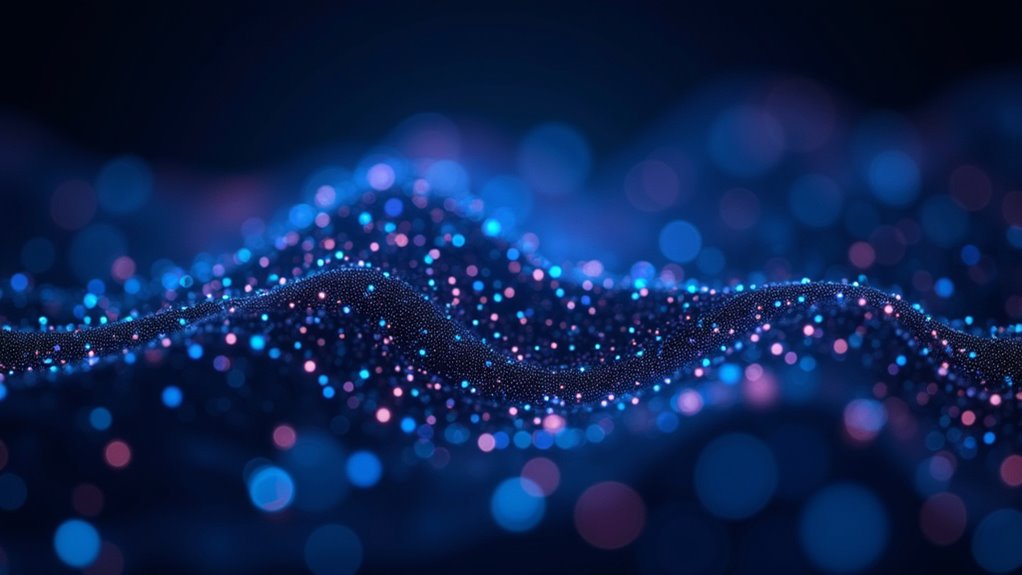
Intelligence, both human and artificial, relies on patterns—and AI models are the digital brains making sense of our data-saturated world. These computational constructs work tirelessly behind the scenes, analyzing countless data points to make predictions and decisions without explicit programming for every scenario. Think of them as super-smart pattern-recognition machines that get better with experience—much like humans, minus the coffee breaks and existential crises.
At their core, AI models are sophisticated algorithms trained through machine learning techniques to identify patterns in data. Neural networks, inspired by the human brain’s structure, allow these models to process complex information through interconnected layers of artificial neurons. AI models utilize different weights & parameters that determine the importance of connections and adjust learning processes during training. Among the most popular models today are Large Language Models that generate human-like text and excel in translation and summarization tasks.
Neural networks: digital minds connecting artificial neurons to see patterns humans might miss.
You’ve likely interacted with these models today without realizing it. That email spam filter? AI model. Netflix recommendations? Also AI models. Your life is surrounded by these invisible digital decision-makers, for better or worse.
The impact of AI models stretches across industries, from healthcare diagnostics to financial fraud detection. They’re the workhorses powering chatbots, facial recognition, and those eerily accurate product recommendations that make you wonder if your phone is eavesdropping. (It’s not—it’s just really good pattern recognition.)
Training these models requires massive datasets and computing resources. Data scientists must clean and prepare information before feeding it to algorithms through supervised, unsupervised, or reinforcement learning methods. The results get tested, tweaked, and tested again until performance meets acceptable standards.
As AI technology advances, foundation models pre-trained on vast datasets are emerging as versatile tools adaptable to multiple applications. The future promises even more sophisticated models with enhanced reasoning capabilities and broader applications. Responsible development of these systems will require thoughtful regulation to balance innovation with ethical considerations about algorithmic bias and privacy.
Understanding AI models isn’t just technical trivia—it’s increasingly essential knowledge as these systems become more deeply integrated into our daily lives, shaping decisions that affect everything from job applications to medical treatments. The algorithms are watching, learning, and deciding—shouldn’t you know how they work?
Frequently Asked Questions
How Do You Measure an AI Model’s Ethical Implications?
Measuring AI ethics isn’t a one-and-done affair. Organizations conduct regular bias assessments to identify unfair outcomes across demographic groups—yes, those algorithms can be prejudiced too!
Fairness evaluation frameworks quantify how equitably systems treat different populations. Ethics audits examine transparency, privacy impacts, and societal consequences.
Remember, numbers never tell the whole story; human judgment remains essential. The process requires continuous monitoring as societal values evolve and new ethical challenges emerge.
No perfect score exists, just ongoing vigilance.
Can AI Models Develop Consciousness or Self-Awareness?
Current AI models lack consciousness despite public perception.
The consciousness debate centers on whether machines can ever truly experience awareness rather than simulate it. Most experts agree today’s AI has self-awareness limits – they process information without understanding it.
While AI can recognize patterns and generate responses that seem self-aware, they don’t possess the neural architecture for consciousness.
Future developments might change this landscape, but for now, AI remains decidedly non-conscious.
What Careers Focus on AI Model Development?
Careers in AI model development are booming, folks!
Data scientists crunch the numbers that make models smart, while machine learning engineers build the algorithms that power everything.
Software developers integrate these models into actual products you use daily.
Research scientists push the boundaries, asking “what if?” to create tomorrow’s breakthroughs.
Want job security? These roles aren’t just hot—they’re practically on fire in today’s tech landscape.
Pick your path and plunge into it!
How Much Energy Do Large AI Models Consume?
Large AI models consume staggering amounts of energy. Training models like GPT-4 can emit greenhouse gases equivalent to hundreds of homes annually, while even simple interactions use 10 times more electricity than standard Google searches.
Energy consumption is directly tied to model size and complexity, and computational demands are doubling every 100 days. By 2030, AI data centers may require an additional 14 GW of power capacity.
Model efficiency improvements are essential to mitigate this growing environmental footprint.
Are There International Regulations Governing AI Model Usage?
Yes, international AI regulations exist but remain fragmented.
The EU leads with its extensive AI Act, while other countries develop their own compliance frameworks. Global standards are emerging through organizations like the Council of Europe, which emphasizes human rights in AI governance.
Companies face a patchwork of ethical guidelines and legal requirements across jurisdictions. This regulatory landscape continues evolving, with most extensive frameworks focusing on high-risk AI applications rather than imposing universal restrictions on all model usage.